Digging beyond Conversion Rate using primary and secondary conversion metrics and avoiding the common testing mistakes
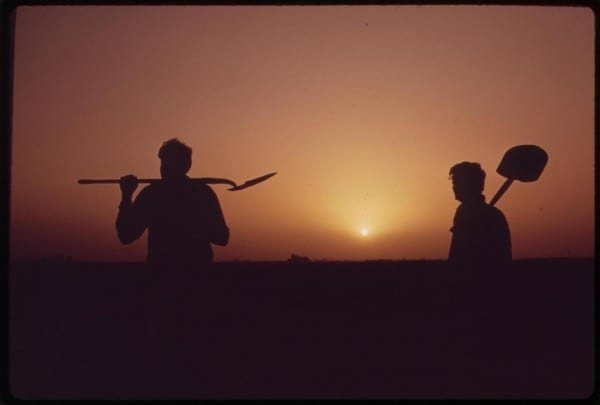
A/B testing is certainly not new, with the number of people and companies involved in testing is continuing to grow at an impressive rate.
Many companies start tentatively with a few sample tests, without investing in expertise or training in how to embed robust testing processes.
Drawing conclusions based on half-cooked tests is a sure-fire way to kill internal faith in your testing programme. You’re also potentially missing out on some of the most interesting insights.
I’ve written before about the importance of using both qualitative and quantitative research to develop the strongest hypotheses for testing. Also the importance of expertise and experience in developing the strongest concepts and then prioritising your testing schedule. However this post will focus primarily on how you then design experiments that accurately track significant changes in user behaviour, some of the common testing pitfalls, and how to get the most insight when interpreting A/B test results.
Tool agnostic caveat
In this article I will refer to Optimizely for testing and Google Analytics, which are our weapons of choice for most clients. However these recommendations and processes are tool agnostic and similar outcomes can be achieved with a number of different tools.
The importance of primary (macro) conversion metrics
When configuring a test we nearly always track (and we need a very good reason not to track) the primary macro conversion for the site. This may be a sale, a subscription or a lead generated. This is the most important site-wide action that aligns with your business goals. It's your most important user goal/KPI.
Without tracking this we may well see an increase in click-through or some other goal but we may just be kicking a problem down the funnel. It’s also important to see if changes in micro conversion (such as a save to wish list for example) effects macro conversion.
An example
We ran a test for a subscription site where we promoted clear pricing information on what was essentially the product page as well as a key landing page template. We found that click-throughs to the subscription page reduced fairly significantly, but the total number of conversions actually increased. We were setting users’ expectations sooner, sending more highly qualified traffic through to the subscription page. In this example, if we hadn’t tracked primary conversion we may have concluded that showing pricing information harmed click-through and should be avoided, when actually it drove an increase in subscriptions.
The value of secondary (micro) conversion metrics
Tracking secondary metrics or “micro conversions” can either be the main goal to track for some tests, or offer another layer of insights to tests where macro conversion is the primary goal.
When designing an experiment we allocate time to consider what additional goals we want to track. It might be click goals for key Call-to-Actions (CTAs) tracked within Optimizely or events for key actions within Google Analytics such as video plays or scroll-depth tracking.
All of this tracking will improve the quality of your leanings. In some cases it can start to provide insights into why a test performed the way it did.
Examples:
- How did conversion vary when users watched the explainer video?
- Did a specific section of tabbed content have an important impact?
- How many people saw the new content that we added in the footer and how did that change their behaviour?
In many cases, the real learning is not simply whether a variation ‘worked’ or not in terms of macro-conversion, but what we can learn about changes in user behaviour which can inspire new hypotheses and influence further tests. You should be constantly trying to build up a picture of our users, their behaviour and which factors are most influential.
Common Test Analysis Pitfalls
I’ve seen a number of articles (as well as grumbling comments) challenging tests presented without a solid statistical basis. While I’ll leave the stats lesson to more qualified statisticians, here are some rules of thumb that have served us really well when testing.
Not enough conversions
When testing, the number of visitors is not nearly as important as the number of conversions of the primary goals of the experiment. Even if you have hundreds of thousands of visitors, if they are not converting then you can’t really learn a lot about the difference between the test variations.
As a rule of thumb we target a minimum of 300* conversions for each variation before we will call a test. I know others who will work with less, and this can be a real challenge for smaller sites or sites without high conversions numbers, but it’s a rule that we stick to rigidly.
Actually this is a bare minimum for us and where possible we try to collect a lot more conversion data. For instance, if we want to drill down into the test results using our analytics tool we will inevitably end up segmenting further as part of our post-test analysis.
For example, if we had 300 conversions for both the control (A) and the variation (B), segmenting by new vs. returning we may now have ~150 in each pot of the four pots. But what if 75% of visitors are new visitors? Each variation might only have 75 conversions for returning visitors. We can very quickly reach a point where our segments are not large enough to lead to significant results.
I can’t underline how valuable large datasets are for detailed post-test analysis.
Testing for short periods (example: weekend peaks)
It may be larger businesses with huge traffic volumes and large numbers of conversions that are particularly guilt of stopping tests too soon. The minimum cycle will vary for each business but for many it will be a week. Running tests for less than a week may mean that you miss out on any daily trends or patterns. For example one of our clients receives 25% of their visits on a Friday and this comes with a change in quality and behaviour. In this case, including or excluding a Friday in a test period could significantly changes the final results.
We recommend running tests for a minimum of two basic business cycles. This allows you to account for weekly trends and makes your conclusions more robust.
Statistical Significance and error bars (example: overlapping error bars)
Experience has taught us to be wary of statistical significance bars within testing tools. We look to achieve a statistical significance of >95% in order to call a test, but only when we have met our criteria for conversions and weekly cycles.
Example:
We have pushed experiments live and then received emails within hours declaring that they have reached statistical significance of >95%. Excitedly logging in to view our test results to find that the number of conversions has barely reached double figures.
Summary
The combination of getting the right number of conversions, minimum testing cycles and statistical significance when used together should allow you to run sound experiments and carry out robust post-test analysis.
Analysing Results in Detail
Basic Analysis
As a minimum for each experiment you should be tracking at least a primary goal within your testing tool and in some cases a number of secondary goals. This will allow you to understand the basic performance of each variation. Nothing too challenging here.
Advanced Analysis – Getting the most from your test
This is where it gets more interesting. Alongside those basic goals you can start to track or simply analyse a much wider set of metrics and dimensions.
Analytics
Pushing custom variables from your testing solution into your analytics tool (this is really simple with Optimizely and Google Analytics) will give you a much wider set of data with which to compare your test variations.
For example:
- Is this test performing differently for new/returning visitors?
- Does a variation work particularly well for a specific traffic source?
- Is a variation performing particularly poorly in a certain browser/OS? Could there be a bug?
- Field level tracking and the tracking of error messages will help you analyse the performance of forms.
Segmentation
Creating custom segments based on your test segments can unlock all of this insights and much, much more. Custom segments for each of your test variations allows you to review the full set of analytics data in order to analyse the impact on user type (new vs returning, traffic sources, average order value, products viewed and bought, etc.)
(Reminder: be careful about sample sizes)
Really clever tricks
Qualitative feedback
Some on-site survey tools will allow you to add test variables to the data collected. This means you can collect some qualitative feedback on your test variations.
For example, you may find that your visitor’s satisfaction rating or NPS changes based on the variations that you test. This could add a completely new angle to the interpretation of your results for an experiment.
Offline conversions
It will likely require a savvy developer but with many testing tools it’s possible to include offline conversion data into your tests (Optimizely info).
This means that you if a visitor sees one of your variations and then converts over the phone you can feed that data in to your test analysis.
Key Take-aways
- Ensure you consider what tracking and goals are important to help you get deeper insights
- Ensure you get the right number of conversions, over a long enough period to be statistically sound and allow for segmented post-test analysis
- Advanced tips: look at pulling in different data sets to add further detail and context to your test results.
Equipped with the recommendations above and the examples of the types of information that you should be tracking you should be all set to avoid common testing pitfalls, collect the right data and carry out more meaningful post-test analysis than.
If you have any other tips or examples please feel free to share in the comments.

Thanks to
Matt Lacey for sharing their advice and opinions in this post. Matt Lacey is Head of Optimisation at
PRWD. You can follow him on
Twitter or connect on
LinkedIn.